Site Map
Homepage
Hurricanes in AGCM
Animations
CLIVAR Hurricane WG EXP.
Climatological Experiments
Inter-annual Experiments
Web Contact: Ming Zhao
Site created with Cheetah.
Last updated: Jun 30 2011
|
Hurricanes in high resolution AGCM
  by   M. Zhao, K. Oouchi, S. Schubert and W. Putman
Motivations and present-day simulations
What controls the global number and distribution of
hurricanes/typhoons and tropical cyclones (TC)? How might these change
with changing climate? The large range of spatial and temporary
scales associated with the physical and dynamical processes of
TC/hurricane genesis makes simple theories difficult. Recent studies
using high resolution global climate models (GCM) offer a promising
approach towards answering these questions (Sugi et. al 2002, McDonald
et. al 2005, Yoshimura et. al 2006, Oouchi et. al 2006, Chauvin et. al
2006, Bengtsson et. al 2007a,b, LaRow et. al 2008, Zhao et. al 2009,
Sugi et. al 2009, Zhao and Held 2010, Held and Zhao 2011). See Knutson
et. al (2010) for a recent review. Despite still relatively coarse
spatial resolution (20-100km), these new GCMs permit a direct
simulation of the generation of TC-like vortices with tropical storm
and hurricane strength. Most of the current TC-permitting GCM
simulations use prescribed sea surface temperature (SST), which do not
allow ocean response and feedback to TCs/hurricanes. Nevertheless,
when forced by observed SST distribution these models did demonstrate
their ability in reproducing many features of TC/hurricane frequency
variability for the past few decades during which reliable
observational data are available. These include the global
geographical distribution, seasonal cycle, interannual variability as
well as decadal trend of storm frequency for multiple ocean
basins. Below shows an example of the simulations from the GFDL HIRAM
(Zhao et. al 2009).

Figure 1. A comparison of geographical distribution of
observed (upper panel) and model simulated (lower panel) hurricane tracks
from 1981 to 2005 . |

Figure 2. Observed and model simulated seasonal cycle (number of
hurricanes per month) for each ocean basin from the 4-member ensemble mean. |
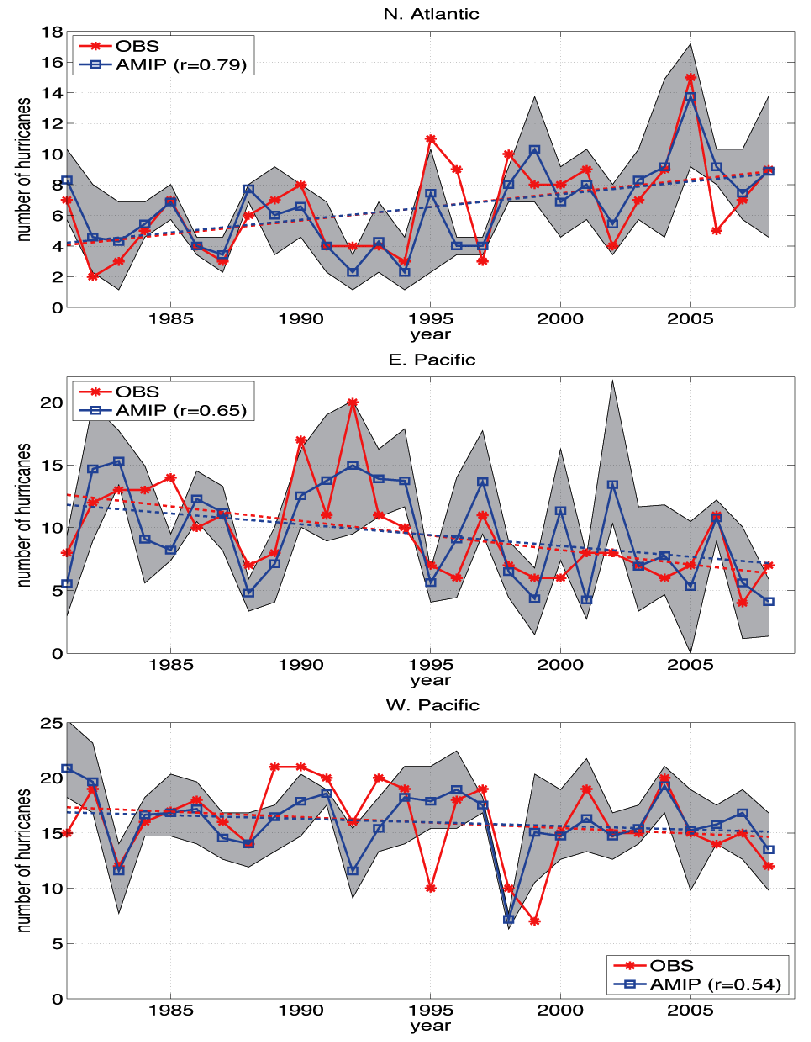
Figure 3. Interannual variation of hurricane count.
Red: observations; blue: model ensemble mean; Model time
series are normalized. Dotted lines show linear trends. |
Hurricane frequency response to global warming
The quality of the present-day simulations has motivated the studies
of TC/hurricane responses to global warming. Most of the GCM studies
in literature utilized a time slice method in which SST warming
anomalies derived from lower resolution coupled climate models are
used as the lower boundary conditions for a global high resolution
atmospheric model. Below displays an example of the simulations based
on the GFDL HIRAM (Zhao et. al 2009, Zhao and Held 2011).
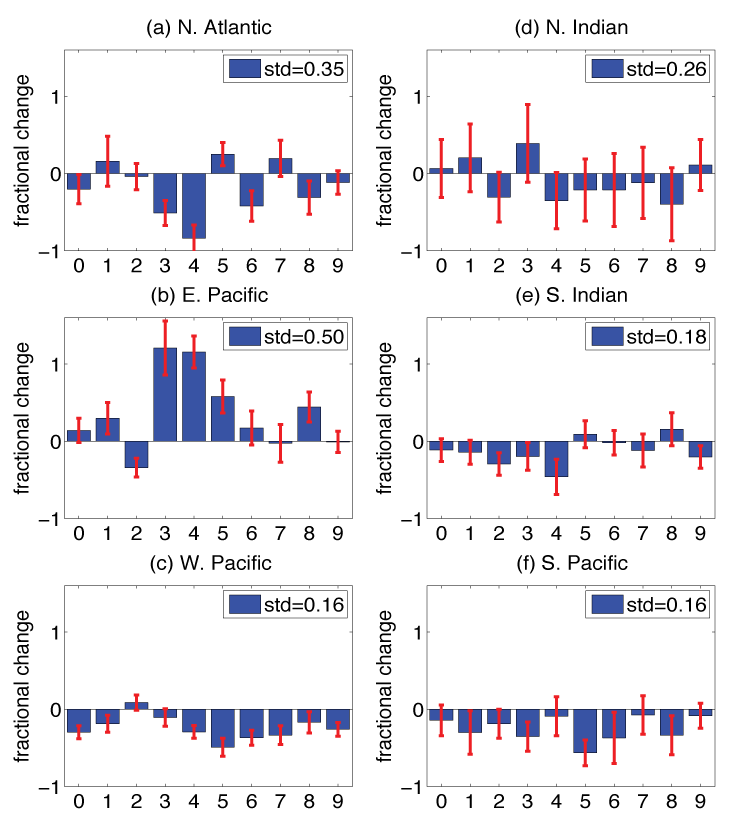
Figure 4. Fractional changes in annual hurricane count for
a) N. Atlantic, b) E. Pacific, c) W. Pacific, d) N. Indian, e) S. Indian, and
f) S. Pacific from 10 SST warming experiments [1-8: 8 individual CMIP3
model projected SST warming anomalies; 0: 18-model ensemble mean
SST anomaly; 9: uniform 2K warming] and the control
experiment. Error bars show the 90% confidence interval; legend
shows the standard deviation of fractional changes across the
models. |
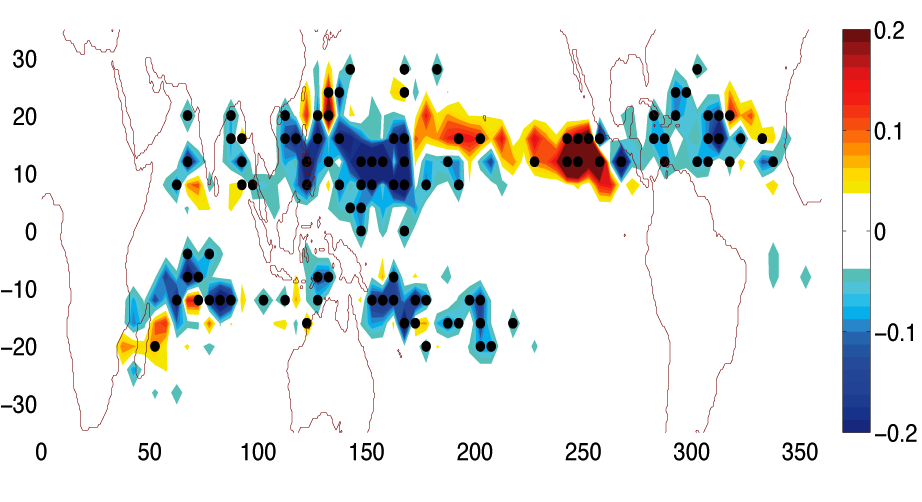
Figure 5. Geographical distribution of the changes in annual
hurricane genesis frequency averaged from the 8 global warming experiments
[unit: number/year per 4x5 (latxlon) degree]. Stippled area denote grid
boxes where at least 6 out of the 8 (75%) models agree on the sign of change. |
Implications, issues and future work through collaborated research
Despite recent progress on high resolution AGCM modeling of
TCs/hurricanes, a number of issues remain that hinder our
understanding and our ability to simulate the variability and change
in TC/hurricane statistics.
- Uncertainties associated with SSTs and other forcings:
For the historical simulations, GFDL HIRAM forced by two different SST
analysis product (HadiSST vs. Reynolds) over the 1981-2008 period
produce large difference in both TC/hurricane trends and the mean
frequency in the North Atlantic. In particular, the observed upward
trend are within the uncertainty of the simulations forced by the
HadiSST (Rayner et. al 2003) while the simulated trend forced by the
Reynolds SST (Reynolds et. al 2002) is much smaller and is not
significantly different from 0. For the future projections, there is
large inter-model spread in the magnitude and (for some basins) even
the sign of the change in hurricane frequency among the different SST
projections in each ocean basin. These differences are due entirely to
the different SST warming patterns. These results further emphasize
the importance in assessing and improving the quality of SST analysis
and its projections in global climate models (Vecchi et. al
2008). However, it is unclear if this strong sensitivity of
TC/hurricane frequency to SSTs is a robust feature across models. It
is also not clear if it is due to the deficiency in models forced by
prescribed SSTs which do not allow ocean response and feedback to
TCs/hurricanes.
- Uncertainties associated with model physics/dynamics:
There are some evidence that the AGCM simulated TC/hurricane
climatology and possibly their response to warming may depend to some
extent on model details in physics/dynamics. For example, in contrast
to the underestimates of W. Pacific storms in Oouchi et. al 2006, the
GFDL HIRAM produce an overestimates of W. Pacific storms (Zhao et. al
2009). It is not clear what cause this difference and how it may
impact the simulated TC/hurricane response to warming. In literature,
however, the differences among simulations often involve simultaneous
changes of both models and forcings. For example, Villarini et. al
(2011) shows some of the divergence in simulated N. Atlantic storm
frequency response among different models for apparently the same SST
forcing are in fact due to the differences in SST forcing since they
are extracted differently (some use linear trends; some use
differencing between the last and the first 20 year period of each
simulation). A systematic model inter-comparison under controlled
conditions through collaborated research such as that proposed here in
US-CLIVAR Hurricane WG would clearly be useful to address these
issues.
- Limitation of models using prescribed SSTs and the time-slice
method:
Despite many years of application of the prescribed
SST method for present-day simulation and time-slice approach for
studying the impact of climate change on weather extremes, some
studies suggest its limitations for studying regional climate change
in certain locations such as the South Asia and the Indian ocean
(Douville et. al 2005, Copsey et. al 2006). Future research would be
needed to better understand these limitations and implications and
develop new methods (e.g., Emanuel et. al 2008, Knutson et. al 2008)
for downscaling the effect of climate change on hurricane statistics
in some regions. Ultimately, fully-coupled ocean atmospheric models
at fine spatial resolution with integrations over centennial scale
will provide the most convincing simulations.
- Hurricane/TC intensity simulation and model resolution:
Most of the current AGCMs still have resolutions too coarse to
simulate the full range of TC intensity distribution. However, TCs at
higher intensity categories such as the major hurricanes account for
most storm damage. Two approaches have been currently utilized to
correcting this deficiency: 1) additional dynamical downscaling (e.g.,
Bender et. al 2010). 2) statistical refinement (e.g., Zhao and Held
2010). Ultimately, we need move to ultra-fine resolution to fully
resolve this issue. Limited ultra-fine resolution model
inter-comparison would be useful to establish an adequate resolution
for this application.
- Uncertainties in TC detection and tracking algorithm
Model simulated TC/hurricane statistics may to some extent be
sensitive to details in TC detection and tracking algorithm. This
often complicates direct comparisons among the different simulation
results in literature. It would be useful to develop a unified TC
detection and tracking scheme for the hurricane-climate
community.
A new approach -- global non-hydrostatic model
Cyclogenesis associated with intra-seasonal disturbances
Along with the traditional approach by hydrostatic models,
non-hydrostatic model, or global cloud-resolving model (GCRM),
has started to set the scene for more accurate simulation and
future projection of tropical cyclone. An advantage of GCRM is
its capability of resolving hierarchy of meso-scale convection
associated with tropical cyclogenesis and intensity
variation. An example of case-study of the cyclone "Nargis" is
presented here. With NICAM (Non-hydrostatic Icosahedral
Atmospheric Model; Satoh et al. 2008; Tomita and Satoh 2004), a
prototype GCRM, an ensemble simulation of cyclone Nargis was
performed with varying the periods of initialization (Taniguchi
et al. 2010). The Nargis made landfall over southern Myanmar on
May 2, 2008 with winds in excess of 65 m/s, over 600 mm total
rainfall, which led to such a tremendous catastrophe across the
Irrawaddy Delta. An observational indicated that Nargis was
initiated in the Bay of Bengal by the emergence of an incipient
cyclonic disturbance as an emanation from a Rossby wave-induced
vortex when the intra-seasonal convective anomaly reached the
Maritime Continent (Kikuchi and Wang, 2010). It was found that
cyclone Nargis formed immediately after the peak of the MJO
signal in the Indian Ocean when the active convective
region associated with the MJO passed through the Bay of Bengal
and was located mainly over the Maritime continent. The cyclone Nargis
developed during the period of northward migration of low-level
zonal wind accompanied with the active convective region
(Fig. 6). Figure 7 indicates that an ensemble of cloud clusters,
a northern branch of the twin disturbances residing across the
equator, develops into the tropical cyclone. Without northward
migration signaling monsoon onset and almost concomitant signal
of the MJO's propagation, no tropical cyclone was formed (with
initial day of April 10). The results suggest that the
representation of northward migration of convection and MJO phase
are important for capturing tropical cyclogenesis in the Bay of
Bengal and that the enhanced predictability of the MJO and the
monsoon onset would be a key factor for the improved prediction
of tropical cyclone. The other simulations with NICAM also
suggest that intra-seasonal disturbance such as MJO is crucial
for formation of tropical cyclone over the basins around the
western Pacific warm pool - across the Indian Ocean to the
western Pacific (Miura et al. 2007;Fudeyasu et al. 2008;Yanase et
al. 2010), sometimes with the combination of large-scale
processes such as a likelihood of tropical upper-tropospheric
trough (Oouchi et al. 2009). For example, Oouchi et al. (2009)
reported an successful simulation of an MJO-associated
cyclogenesis about 3 weeks after the start of the time
integration. The importance of MJO in prediction of tropical
cyclone has also been intensely investigated in ECMWF (Vitart
2009).
Future projection
Computationally demanding GCRM is still not sufficient to perform
many months and years of time integration that can derive
statistically robust future projection of tropical cyclone. Yet, we
would come up with a solution and new collaboration with the
traditional AGCM research with the advent of powerful
computational resources. A first challenge of GCRM to give an
insight into future change of tropical cyclone was reported by
Yamada et al. (2010) from a suite of 14-km mesh time-slice
experiments with NICAM. They discussed possible change in the
cloud height of tropical cyclones as well as the general
statistics of frequency, intensity, and environmental factors for
TCs. A highlight of the study is on the projection of the change
in the cloud height of TC (Fig.8). It was found that the cloud
height becomes taller for more intense TCs and that this
relationship is strengthened with a global-warming condition. This
is the type of the problem that was unable to be developed by
traditional hydrostatic models, and points to an attractive
direction of research with GCRM. An extension of this type of
future projection research with GCRM will be not only appealing on
its own right, but important to help mitigate the uncertainties in
the traditional GCM in projecting future change of tropical cyclone.

Figure 6. Temporal evolution of the observed (re-analysis)
field of zonally averaged zonal wind (m/s) at 850 hPa (JMA/JCDAS) over 80E-100E
(a), blended zonally averaged infrared (IR) brightness temperature (NCEP/CPC
4 km Global IR datasets) shown by the white-navy blue scale (K) over 80E-100E,
and zonally averaged surface precipitation rate (mm/h) (Global Rainfall Map
derived from the JAXA/EORC GSMaP Near Real-Time System, ver. 1.0) shown
by the color scale for values greater than 0.3 (mm/h) over 80E-100E (b) during
the period from April 1 to May 31, 2008. Simulated zonally averaged zonal
wind (m/s) at 850 hPa over 80E-100E (c, e, g) and simulated zonally averaged
outgoing long wave radiation (OLR) (W/m2) shown by the white-navy blue scale
and surface precipitation rate (mm/h) shown by the rainbow color scale for
values grater than 0.3 (mm/h) over 80E-100E (d, f, h).
Panels (c)-(d), (e)-(f), and (g)-(h) show the results of simulations with
initial days of April 10, April 23, and April 24, 2008, respectively. The weather
symbol and double solid circles in each figure show the observed and simulated
position and day of the genesis of cyclone Nargis, respectively. |
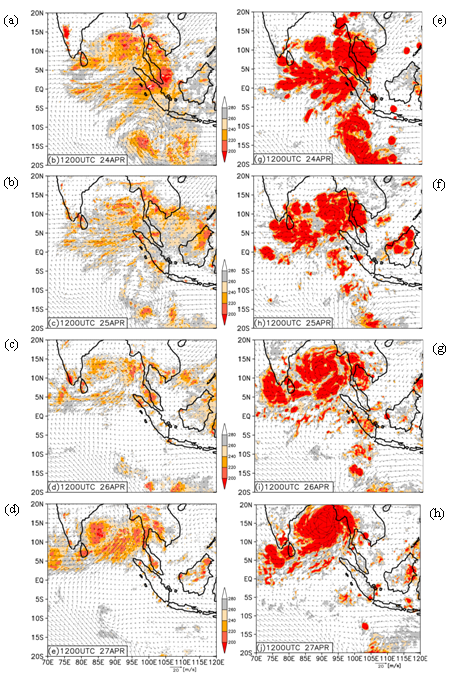
Figure 7. Temporal-spatial evolution of initial disturbances
and tropical depressions from 1200 UTC on April 24-April 27. Blended sea surface
winds at 10 m are shown by vectors (m/s) and infrared (IR) brightness temperature
is shown by the color scale (K, observations) and OLR (W/m2, simulations). Left
column shows observed (re-analysis) fields obtained by JMA/JCDAS (wind) and
NCEP/CPC 4 km Global IR datasets. Right column shows the one of the results of
NICAM simulations (initial day of April 23 case). Observed and simulated times
are shown above each figure. The color scale differs between observed and
simulated data. The 1st, 2nd, 3rd, and 4th rows (from the upper) show the case
of 12:00 UTC on April 24-April 27, respectively.
|

Figure 8. Scatter plots for the cloud top height
and the MSP for the simulated TCs. CTL (blue inverted triangle) and
GW (red triangle). The regression line for CTL (blue broken line)
and GW (red solid line) is f(x) = 32.8-0.0158x, and g(x) =
42.5-0.0253x, respectively, where x denotes MSP [hPa]. The cloud
height is defined as the highest altitude below which the sum of the
solid hydrometeors (snow, cloud ice, and water contents) exceeds
1.0x10.5 kg/kg at least for 30 grid points in 10x10degree
box.
| 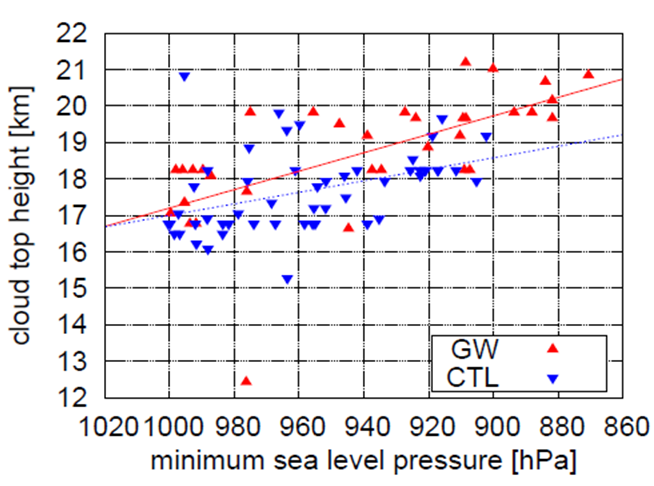
|
NASA GEOS-5 Global High-Resolution Hurricane Simulations
Current NWP is at the limit of hydrostatic models, with resolutions of
10- to 20-km. Results from a global non-hydrostatic version of the
NASA Goddard Earth Observing System global atmospheric model version 5
(GEOS-5) hint at the potential for improved numerical weather
prediction skill as we advance to non-hydrostatic models at
resolutions finer than 14-km. The main benefits will likely be in the
tropics, with better predictions of the genesis stages of tropical
cyclones and of the internal structure of their mature
stages. Inter-seasonal variability of tropical cyclone activity in
terms of both frequency and intensity can now be explored with a
high-resolution global climate model.
Hurricane Bill 2009
10-day NWP experiments at 3.5-, 7-, and 28-km initialized 16-Aug-2009
at 21z from a 50-km GEOS-5 analysis develop tropical storm Bill to a
category 4 hurricane before weakening by the end of the forecast
period (Movie 1). Comparing
OLR from 28- and 3.5-km GEOS-5 forecasts with 4-km global-merged IR
brightness temperature data, show the improved organization of
convection throughout the tropics and within the circulation of
hurricane Bill as resolution increases (Figure 9).
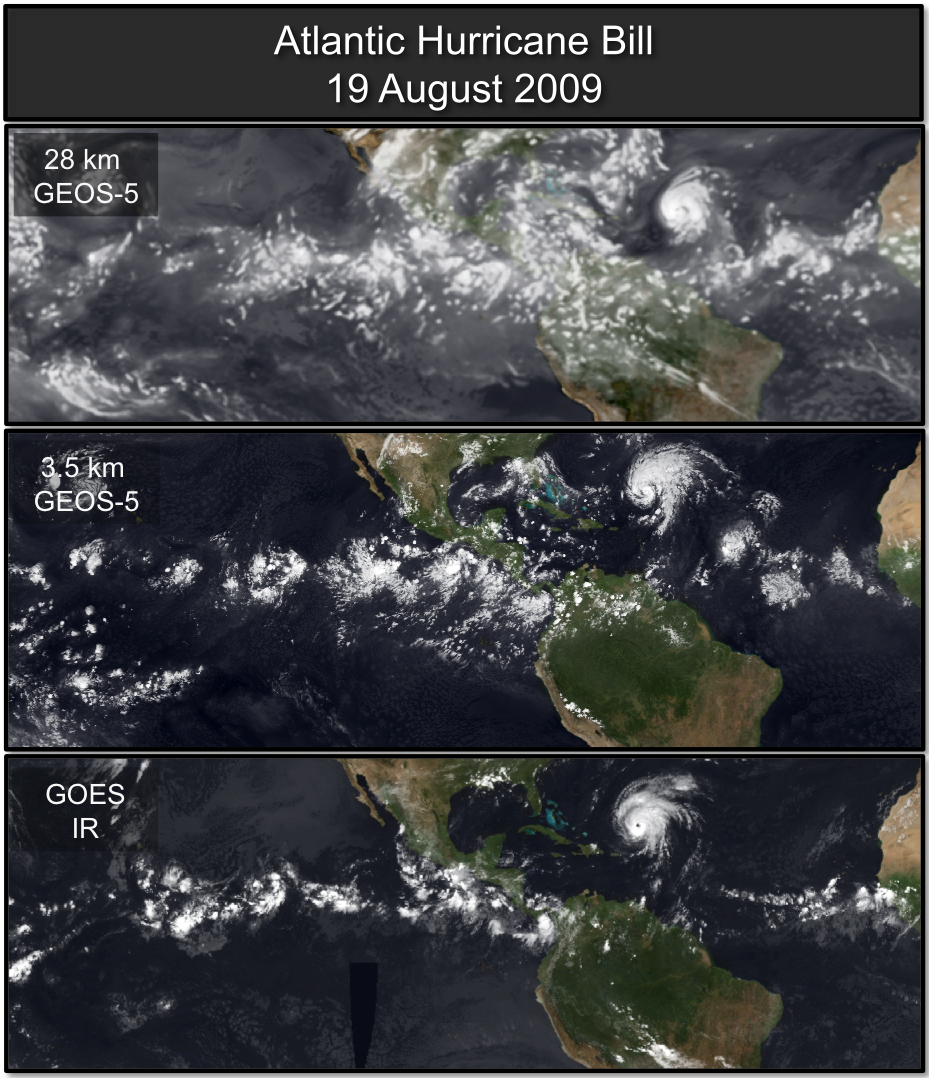
Figure 9. Composite GOES infrared satellite
imagery (bottom), OLR from GEOS-5 at 3.5-km (middle) and 28-km (top)
as seen on 20-August-2009 at 18z, 69 hours into the 15-day forecasts
with GEOS-5.. |
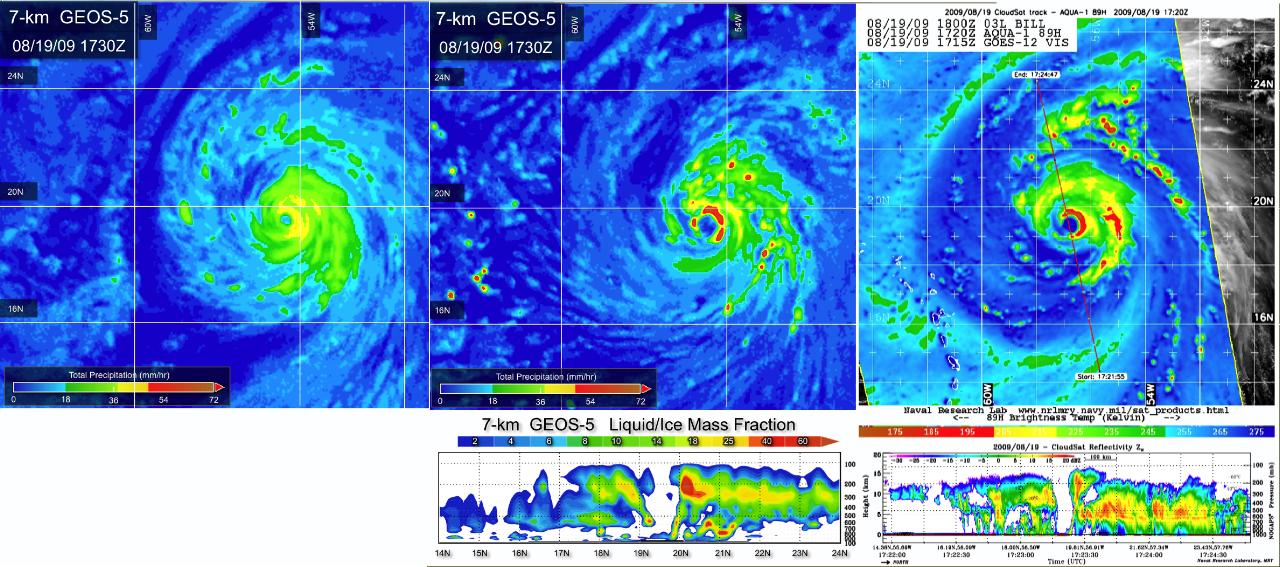
Figure 10. NASA's satellite CloudSat
captured an eye overpass of category 4 Hurricane Bill on
19-August-2009 at 17:20z (bottom-right), the 89 GHz AMSR-E instrument
onboard the Aqua spacecraft (top-right) reveals deep convection and
low brightness temperatures ( < -117 F) as shown by the bright red
and deep brown colors (Image Credits: NASA/JPL/The Cooperative
Institute for Research in the Atmosphere (CIRA), Colorado State
University). Precipitation intensities from GEOS-5 at 7-km with the
full influence of the RAS convection scheme (top-left) produces
reduced convection and a compact drizzle filled eye. Precipitation
rates from GEOS-5 at 7-km (top-center) with the reduced influence of
RAS by the Tokioka limiter mirror the horizontal structure of deep
convection as observed by the AMSR-E instrument, while a vertical
cross-section of liquid and ice fractions from GEOS-5 (bottom-center)
depicts the well defined eye-wall and deep thunderstorms in the
surrounding convection. |
The non-hydrostatic implementation of GEOS-5 executes with the same
physics as the hydrostatic implementation. However, the relaxed
Arakawa-Schubert convective parameterization [Moorthi and Suarez,
1992] is modified to become a non-precipitating shallow convection
scheme by use of a stochastic Tokioka constraint. This constraint
selectively suppresses the convection scheme by placing a random lower
limit on the plume entrainment at resolutions capable of dynamically
resolving convection [Tokioka et al, 1998]. This has a dramatic impact
on the representation of convection within the developing hurricane at
increasing resolutions. Comparing precipitation rates from 7-km
GEOS-5 forecasts with observed brightness temperature from AMSR-E as
an indication of intense convective activity within the hurricane
eye-wall and outer rain-bands (Figure 10) reveals the impact of the
reduced role of convective parameterization. With the full influence
of the RAS convection scheme (Figure 10, left) precipitation
intensities are greatly reduced, the eye-wall is too compact and
filled with shallow convection and light drizzle. Reducing the role
of RAS through the Tokioka limiter (Figure 10, center), permits intense
precipitation to form within the eye-wall and surrounding rain-bands,
the diameter of the eye becomes representative of the observations
(Figure 10, right) and clear of significant drizzle. The vertical
distribution of liquid and ice fractions from GEOS-5 (Figure 10,
bottom-center) show a well-defined eye wall, with deep convection and
ice cloud formation at the 200-300 hPa levels as observed by CloudSat
reflectivity (Figure 10 bottom-right).
Nature Runs
Building on the improved representation of convection and tropical
cyclone structure, GEOS-5 has been used to prepare "Nature Runs" at
14-km globally. These AMIP-style "Nature Runs" are free running
global simulations initialized from 50-km analyses and forced only by
observed sea surface temperatures and climatological aerosol emissions
(Movie 2). GEOS-5 demonstrates
a capability to predict realistic occurrences of tropical cyclone
activity in terms of frequency and track location in all basins
(Figure 11). The 2005 Atlantic basin activity reflects the unusually
high number of storms for the period from May-Dec, as GEOS-5 simulates
25 storms compared with the 27 observed.
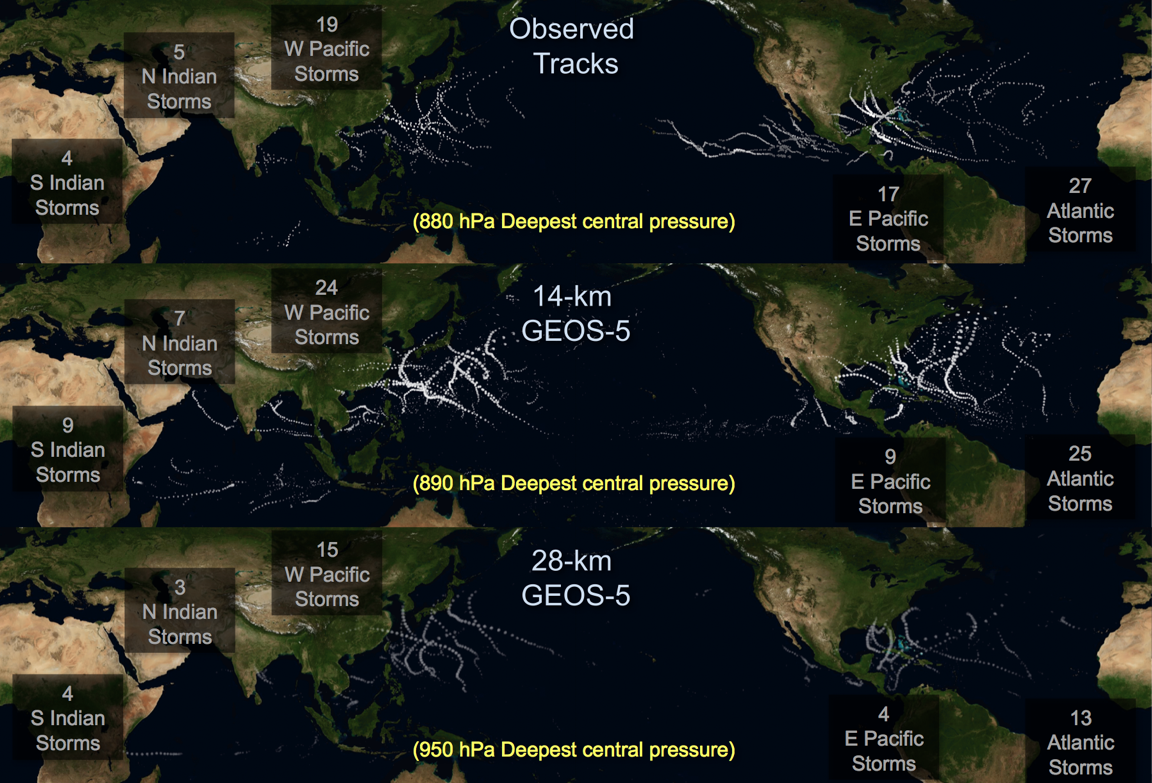
Figure 11. Global tropical cyclone
tracks from May through December 2005 as observed (top), and
simulated in nature runs with GEOS-5 at 14-km (middle) and 28-km
(bottom). Counts of total tropical cyclones for each basin are
presented along with the minimum value of observed/modeled sea level
pressure over all storms. |
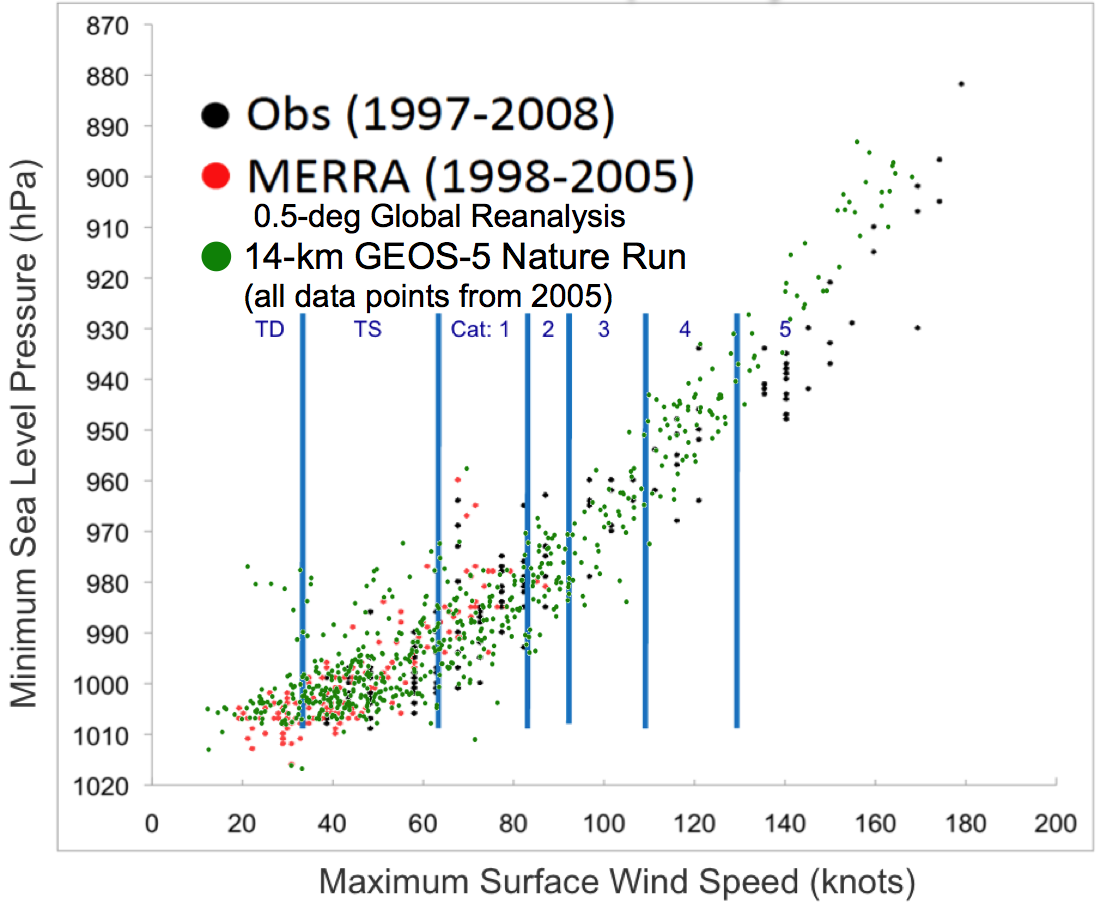
Figure 12. Minimum sea level
pressure and maximum near surface winds as observed in all Atlantic
tropical cyclones from 1997-2008 (black circles), for all Atlantic
tropical cyclones from MERRA (a 50-km re-analysis using GEOS-5 from
1998-2005, red circles), and for all 3-hourly observed Atlantic
tropical cyclones from a 14-km nature run with GEOS-5 (green
circles). |
The distribution of minimum central pressure and maximum near surface
wind speeds from GEOS-5 reflects a distribution similar to
observations for all storms from 1997 to 2008 (Figure 12). The deepest
central pressure modeled by GEOS-5 was 890 hPa in an Atlantic
hurricane, compared with 880 hPa in the observations, and the maximum
winds modeled reach in excess of 160 knots. This marks a substantial
improvement from the MERRA reanalysis performed with GEOS-5 at 50-km
resolution, where the maximum intensity for Atlantic hurricanes
reaches only category 2 strength.
References
- Bender, M., T. Knutson, R. Tuleya, J. sirutis,
G. Vecchi, S.T.Garner and I. Held, 2010: Modeled impact of
anthropogenic warming on the frequency of intense Atlantic
hurricanes. Science 327, 454–458.
- Bengtsson, L., K. Hodges and M. Esch, 2007a:
Tropical cyclones in a T159 resolution global climate model:
comparison with observations and re-analyses. Tellus, 59A,
396–416.
- Bengtsson, L., K. Hodges, M. Esch, N. Keenlyside,
L. Kornblueh, J.-J. Luo, and T. Yamagata, 2007b: How may tropical
cyclones change in a warmer climate. Tellus, 59A, 539–561.
- Chauvin, F., J.-F. Royer, and M. Deque, 2006:
Response of hurricane-type vortices to global warming as simulated by
ARPEGE-climat at high resolution. Clim. Dyn., 27,
377–399.
- Copsey, D., R. Sutton, and J. Knight, 2006: Recent trends in sea level pressure
in the indian ocean region. Geophys. Res. Let., 33,
L19, 712, doi:10.1029/2006GL027, 175.
- Douville, H., 2005: Limitations of time-slice experiments for predicting
regional climate change over South Asia. Climate Dynamics, 24, 373–391.
- Emanuel, K., R. Sundararajan, and J. Williams, 2008: Hurricanes and global
warming: results from downscaling IPCC AR4 simulations. Bull. Amer. Meteor. Soc.,
347–367.
- Fudeyasu, H, Y. Wang, M. Satoh, T. Nasuno, H. Miura, W. Yanase, 2008:The global
cloud-system-resolving model NICAM successfully simulated the lifecycles of two
real tropical cyclones. Geophys. Res. Lett. 35: L22808,
doi:10.1029/2008GL0360033
- Gualdi, S., E. Scoccimarro, A. Navarra, 2008:
Changes in Tropical Cyclone Activity due to Global Warming: Results
from a High-Resolution Coupled General Circulation
Model. J. of Clim., 21, 5204–5228.
- Held, I. M. and M. Zhao, 2011: The response of
tropical cyclone statistics to an increase in CO2 with fixed sea
surface temperatures. J. Climate, in press.
- Kikuchi, K, and B. Wang, 2010:
Formation of tropical cyclones in the Northern Indian
Ocean associated with two types of tropical intraseasonal oscillation
modes. J.Meteor.Soc.Japan,88,475–496.
- Knutson, T., J. Sirutis, S. Garner, G. Vecchi, and
I. Held, 2008: Simulated reduction in Atlantic hurricane frequency
under twenty-first-century warming condition. Nature Geoscience,
doi:10.1038/ngeo202.
- Knutson, T., et al., 2010: Tropical cyclones and
climate change. Nature Geoscience, doi:10.1038/ngeo0779.
- LaRow, T., Y.-K. Lim, D. Shin, E. Chassignet, and
S. Cocke, 2008: Atlantic basin seasonal hurricane
simulations. J. Climate, 21, 3191–3206.
- Li, T., M. Kwon, M. Zhao, J.-S. Kug, J.-J. Luo, and
W. Yu, 2010: Global warming shifts Pacific tropical cyclone
location. Geophys. Res. Let., 37, L21, 804, doi:10.1029/2010GL045,124.
- McDonald, R., D. Bleaken, D. Creswell, V. Pope, and
C. Senior, 2005: Tropical storms: representation and diagnosis in
climate models and the impact of climate change. Clim. Dyn.,
25, 19–36.
- Miura, H, M. Satoh, T. Nasuno, A.T. Noda, and K. Oouchi, 2007:
An Madden-Julian Oscillation event simulated using a global cloud-resolving model.
Science, 318,1763–1765.
- Moorthi, S, and M. J. Suarez, 1992:
Relaxed Arakawa-Schubert: A parameterization of moist convection for general circulation
models. Mon. Wea. Rev., 120, 978–1002.
- Oouchi, K., J. Yoshimura, H. Yoshimura, R. Mizuta,
S. Kusunoki, and A. Noda, 2006: Tropical cyclone climatology in a
global-warming climate as simulated in a 20 km mesh global atmospheric
model: Frequency and wind intensity. J. Meteor. Soc. Japan,
84, 259–276.
- Oouchi, K., A.T. Noda, M. Satoh, H. Miura, H. Tomita.
T. Nasuno, and S. Iga, 2009: A simulated preconditioning of typhoon
genesis controlled by a boreal summer Madden-Julian Oscillation
event in a global cloud-system-resolving model. SOLA, 5,
065–068, doi:10.2151/sola.2009-017.
- Rayner, R., D. Parker, E. Horton, C. Folland,
L. Alexander, and D. Rowel, 2003: Global analyses of sea surface
temperature, sea ice, and night marine air temperature since the late
nineteenth century. J. Geophys. Res., 108, D14,4407,doi:10.1029/2002JD002,670.
- Reynolds, R., N. Rayner, T. Smith, D. Stokes and
W. Wang, 2002: An improved in situ and satellite SST analysis for
climate. J. Climate, 15, 1609–1625.
- Satoh, M, T. Matsuno H, Tomita, H, Miura, T. Nasuno and S. Iga S, 2008: Nonhydrostatic Icosahedral Atmospheric Model (NICAM) for global cloud resolving simulations.
J. Comput. Phys. 227: 3486–3514, doi:10.1016/j.jcp.2007.02.006
- Sugi, M., A. Noda, and N. Sato, 2002: Influence of
the global warming on tropical cyclone climatology: An experiment with
the JMA global model. J. Meteor. Soc. Japan, 80,
249–272.
- Sugi, M., H. Murakami, and J. Yoshimura, 2009: A
reduction in global tropical cyclone frequency due to global
warming. SOLA, 5, 164–167.
- Taniguchi, H., W. Yanase, and M. Satoh, 2010: Ensemble
simulation of cyclone Nargis by a global cloud-system-resolving model -
modulation of cyclogenesis by the Madden-Julian Oscillation. J. Meteor. Soc. Japan,88,
571–591.
- Tomita, H. and M. Satoh, 2004: A new dynamical framework of
nonhydrostatic global model using the icosahedral grid. Fluid Dyn. Res.,34,
357–400.
- Vecchi, G. and B. Soden, 2007: Effect of remote sea surface temperature change
on tropical cyclone potential intensity. Nature, 450,
1066–1071 doi:10.1038 Nature, 423.
- Vecchi, G., B. Soden, A. Wittenberg, I. Held, A. Leetmaa, and M. harrison,
2006: Weakening of tropical pacific atmospheric circulation due to
anthropogenic forcing. Nature, 441, 73–76.
- Vecchi, G., K. Swanson, and B. Soden, 2008: Whither hurricane activity?
Science, 322, 687–689.
- Villarini, G., G. Vecchi, T. Knutson, M. Zhao, and J. Smith, 2011: North
Atlantic tropical storm frequency response to anthropogenic forcing:
projections and sources of uncertainty. J. Climate, in
press.
- Vitart, F, 2009:
Impact of the Madden Julian Oscillation on tropical storms and risk
of landfall in the ECMWF forecast system. Geophys. Res. Lett. ,36:
L15802, doi:10.1029/2009GL039089.
- Yamada, Y, K. Oouchi, M. Satoh, H. Tomita, and W. Yanase,
2010: Projection of changes in tropical cyclone activity and cloud
height due to greenhouse warming: global cloud-system-resolving approach.
Geophys. Res. Lett., 37, L07709, doi:10.1029/2010GL042518.
-- "Research Highlights" in Nature, Apr 22 (2010) doi:10.1038/4641107c,
Climate change: Fewer, taller, fiercer, Nature, 464, 1107.
- Yanase, W, H. Taniguchi, and M. Satoh M, 2010:
Environmental modulation and numerical predictability associated with the genesis
of tropical cyclone Nargis (2008). J. Meteor. Soc. Japan,88, 497–519.
- Yoshimura, J. and M. Sugi, 2005: Tropical cyclone
climatology in a high-resolution AGCM - Impacts of SST warming and CO2
increase. SOLA, 1, 133–136, doi:10.2151.
- Zhao, M., I. M. Held, S.-J. Lin, and G. A. Vecchi,
2009: Simulations of global hurricane climatology, interannual
variability, and response to global warming using a 50km resolution
gcm. J. Climate, 22, 6653–6678.
- Zhao, M., I.M. Held and G.A. Vecchi, 2010:
Retrospective forecasts of the hurricane season using a global
atmospheric model assuming persistence of SST
anomalies. Mon. Wea. Rev., 138, 3858–3868.
- Zhao, M. and I.M. Held, 2010: An analysis of the
effect of global warming on the intensity of Atlantic hurricanes using
a GCM with statistical refinement. J. Climate, 23,
6382–6393.
- Zhao, M. and I. M. Held, 2011: TC-permitting GCM
simulations of hurricane frequency response to sea surface temperature
anomalies projected for the late 21st century. J. Climate,
in press.
- Zhao, M., I. M. Held and S-J Lin, 2011: Some
counter-intuitive dependencies of tropical cyclone frequency on
parameters in a GCM. J. Atmos. Sci., submitted.
|